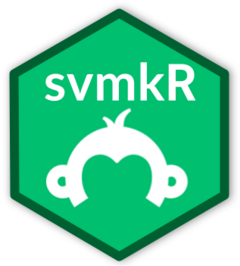
Format and save banner object to file
write_banners.Rd
Take the output from a call to make_banners
, populate a spreadsheet with the banner data, format/stylize it in the SurveyMonkey research format, and save it locally. Currently allows for up to one level of nesting in columns.
Usage
write_banners(
banners.output,
title = "Main",
file.path,
file.overwrite = TRUE,
file.append = FALSE,
drive.folder.path = NULL,
drive.overwrite = TRUE,
tab.name = "Main",
logo = NULL,
include.moe = TRUE,
include.dates = TRUE,
date.var = "response_date",
weight.var = NULL,
...
)
Arguments
output list from a call to
make_banners()
.- title
title of the tab/sheet.
- file.path
local file path to save banners as
.xlsx
file.- file.overwrite
whether or not to overwrite the file specified at
file.path
; otherwise keep both and update the file path.- file.append
whether or not to append the current tab to the file specified at
file.path
if it exists; if there is already a tab with the name specified, update the tab name.- tab.name
name of the tab/sheet to populate.
- include.moe
whether or not to include the Margin of Error for the survey in the banner headers.
- include.dates
whether or not to include the date range for survey responses in the banner headers.
- date.var
if
include.dates
is set toTRUE
, the date variable to look for in the data used to createbanners.output
.- weight.var
the column in the data used to create
banners.output
corresponding to weights for the Margin of Error estimate.
Examples
data(ev22)
if (FALSE) { ## not run
# Prepare data for banners
ev22.coded = ev22 %>%
let(
## code/label categorical variables
age3 = recode(age,
"18-34" = 18 %thru% 34 ~ 1,
"35-64" = 35 %thru% 64 ~ 2,
"65+" = 65 %thru% hi ~ 3),
gender = recode(gender,
"Male" = 1 ~ 1,
"Female" = 2 ~ 2),
income3 = recode(as.numeric(income),
"<$50k" = 1 %thru% 3 ~ 1,
"$50k-100k" = 4 %thru% 5 ~ 2,
">$100k" = 6 %thru% 7 ~ 3,
"No answer" = 8 ~ 4),
educ2 = recode(as.numeric(education),
"<HS-College" = 1 %thru% 4 ~ 1,
">College" = 5 %thru% 6 ~ 2),
purchase = recode(purchase,
"Purchased new" = 1 ~ 1,
"Purchased used (non-certified pre-owned)" = 2 ~ 2,
"Purchased used (certified pre-owned)" = 3 ~ 3,
"Leased new" = 4 ~ 4,
"Leased used" = 5 ~ 5,
"Other" = 6 ~ 6),
vehage = recode(vehage,
"Less than 1 year old" = 1 ~ 1,
"1 - 2 years old" = 2 ~ 2,
"3 - 5 years old" = 3 ~ 3,
"6 - 10 years old" = 4 ~ 4,
"More than 10 years old" = 5 ~ 5),
vehcost = recode(vehcost,
"Under $5,000" = 1 ~ 1,
"$5,000 - $9,999" = 2 ~ 2,
"$10,000 - $19,999" = 3 ~ 3,
"$20,000 - $29,999" = 4 ~ 4,
"$30,000 - $39,999" = 5 ~ 5,
"$40,000 - $49,999" = 6 ~ 6,
"$50,000 - $59,999" = 7 ~ 7,
"$60,000 or more" = 8 ~ 8),
## label multi-response variables
## note: make sure to re-code dichotomous "one-hot encoded" columns into distinct level
ev_heard_1 = recode(ev_heard_1, "Audi e-tron" = 1 ~ 1),
ev_heard_2 = recode(ev_heard_2, "BMW i3" = 1 ~ 2),
ev_heard_3 = recode(ev_heard_3, "Cadillac LYRIQ" = 1 ~ 3),
ev_heard_4 = recode(ev_heard_4, "Chevrolet Bolt" = 1 ~ 4),
ev_heard_5 = recode(ev_heard_5, "Faraday Future" = 1 ~ 5),
ev_heard_6 = recode(ev_heard_6, "Fisker" = 1 ~ 6),
ev_heard_7 = recode(ev_heard_7, "Hyundai Electric" = 1 ~ 7),
ev_heard_8 = recode(ev_heard_8, "Jaguar I-Pace" = 1 ~ 8),
ev_heard_9 = recode(ev_heard_9, "Kia Niro EV" = 1 ~ 9),
ev_heard_10 = recode(ev_heard_10, "Lucid Motors" = 1 ~ 10),
mech_factors_1 = recode(mech_factors_1, "Price / affordability" = 1 ~ 1),
mech_factors_2 = recode(mech_factors_2, "Convenience" = 1 ~ 2),
mech_factors_3 = recode(mech_factors_3, "Trust / relationship" = 1 ~ 3),
mech_factors_4 = recode(mech_factors_4, "Availability" = 1 ~ 4),
sub_services_1 = recode(sub_services_1, "In-car internet" = 1 ~ 1),
sub_services_2 = recode(sub_services_1, "Remote car start capability" = 1 ~ 2),
sub_services_3 = recode(sub_services_1, "Live traffic / navigation" = 1 ~ 3),
sub_services_4 = recode(sub_services_1, "In-vehicle safety and security" = 1 ~ 4),
sub_services_5 = recode(sub_services_1, "Multiple driver profiles" = 1 ~ 5),
sub_services_6 = recode(sub_services_1, "Cloud dash cam monitoring" = 1 ~ 6),
sub_services_7 = recode(sub_services_1, "Car software updates" = 1 ~ 7),
sub_services_8 = recode(sub_services_1, "AI- and self-driving capabilities" = 1 ~ 8),
sub_services_9 = recode(sub_services_1, "Car-only media and streaming services" = 1 ~ 9),
sub_services_10 = recode(sub_services_1, "Heated seats" = 1 ~ 10)
) %>%
apply_labels(gender = "Gender",
age3 = "Age: 3 categories",
educ2 = "Education",
income3 = "Income",
vehage = "How old is your main vehicle?",
vehcost = "In total, how much did you pay to buy or lease your main vehicle?",
purchase = "How did you purchase or lease your main vehicle?",
## note: for multiresponse variables, only need to name one column
ev_heard_1 = "Which of the following electric vehicles have you heard of?",
mech_factors_1 = "Select the top 3 factors that are most important to you when selecting an auto repair provider:",
sub_services_1 = "Which of the following services would you be willing to pay a recurring subscription for in a car?")
# Make and save banners
ev22.banners <- ev22.coded %>%
make_banners(row.vars = list("purchase","vehage","ev_heard*",c("mech_factors_1","mech_factors_2","mech_factors_3","mech_factors_4")),
col.vars = list("age3","educ2",c("sub_services_1","sub_services_2","sub_services_3","sub_services_4")),
weight.var = "weight_genpop",
date.var = "response_date",
total.row.position = "below",
preview = TRUE) %>%
write_banners(file.path = "ev22.xlsx",
file.overwrite = TRUE,
title = "Electric Vehicles Study",
logo = "mntv") %>%
upload_banners(drive.overwrite = TRUE,
drive.file.name = "EVs Crosstabs",
drive.folder.path = "https://drive.google.com/drive/u/1/folders/XXXX")
}